Cognition and learning for robots
Learning from their own experience is an important ability for robots working in a complex daily environment. We attempt to describe the essential mechanism of developing cognitive abilities through the robot's own sensory-motor experience. To achieve this, we are conducting interdisciplinary studies by taking a synthetic approach in experiments on neural network modeling and robotics.
Integrating Object Manipulation Behaviors into the Attractor Dynamics (2005 - )
This is part of a collaborative research project with RIKEN Brain Science Institute.
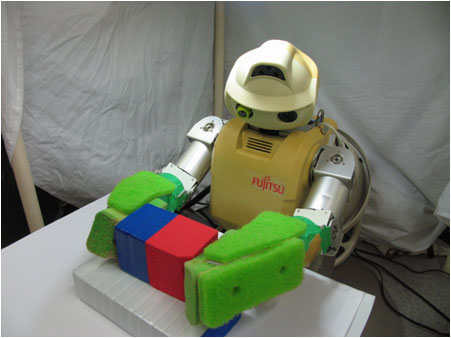
We are exploring the problems of symbols and behavior generation. Although in conventional cognitive science, symbol representations and their manipulations are assumed as a priori, a crucial question is to what degree such symbol systems can be realized in neuronal dynamical systems. It is also important to ask how they can be acquired as grounded to sensory-motor experiences. We are investigating these problems by taking a synthetic approach using neural network modeling and robotics experiments.
Developmental Learning of Motor Control System (2005 – 2008)
This was part of collaborative research done with RIKEN Brain Science Institute.
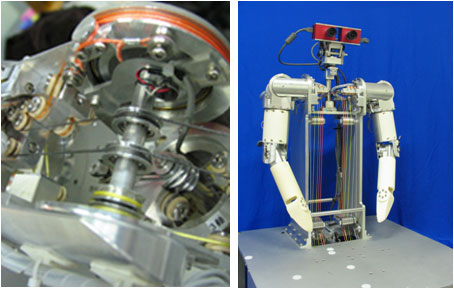
Humans learn skilled behavior through repetition of our own behavior experiences. From a brain science perspective, we speculate that such skilled behaviors might be acquired in the inferior parietal lobe through the repeated sensory-motor experiences, and we therefore propose a brain model. We utilized two neural networks in our study. One was a continuous time recurrent neural network (CTRNN), which learns the trajectory of joint angles to achieve the task. The other was a recurrent neural network (RNN), which learns the relation of muscle activity to joint angles. We tested the proposed model by applying it to the task of reaching for an object using a real robot with a tendon-based actuation mechanism.